
Mitigating Main Bearing Failure in Wind Turbines
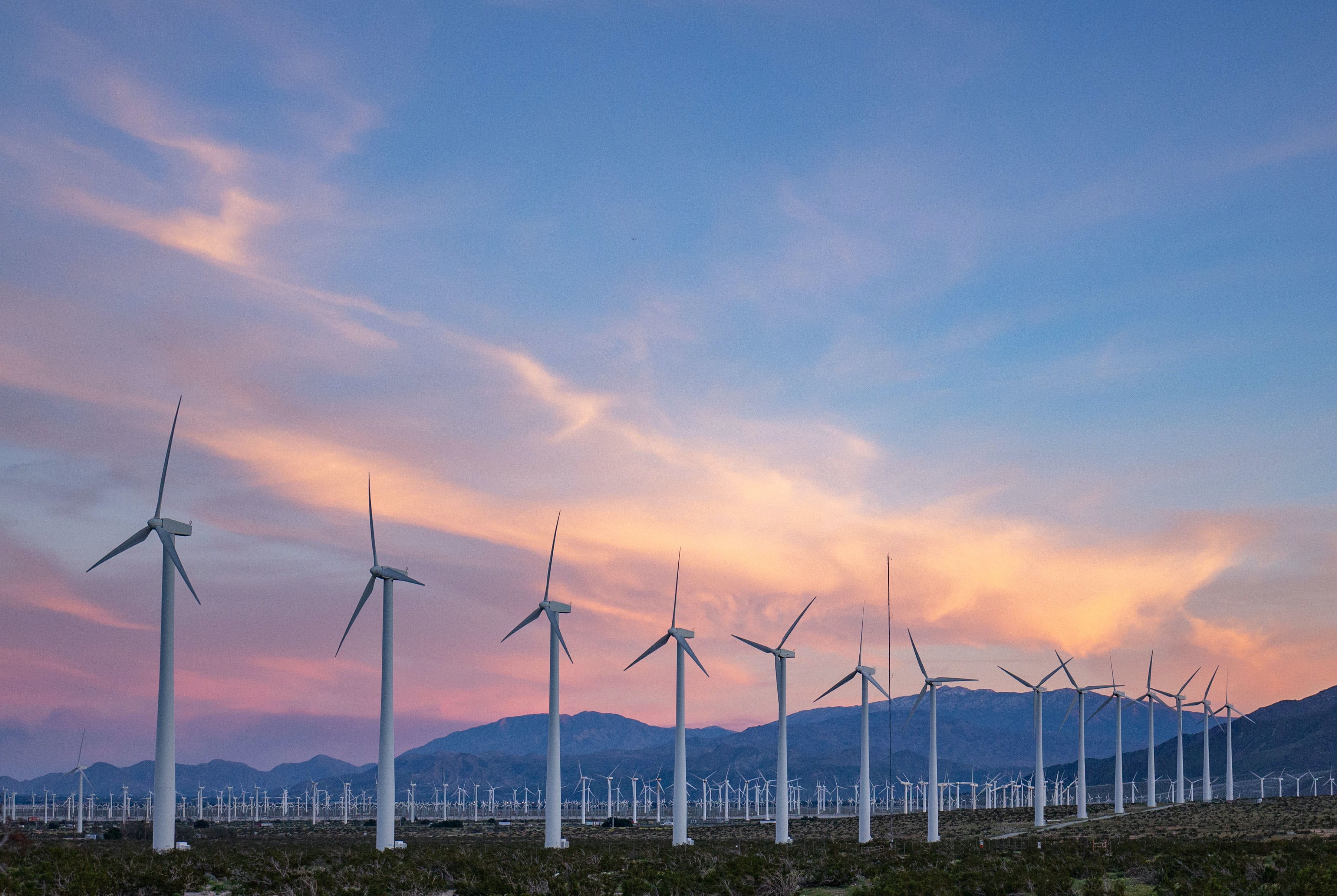
In the quest for sustainable energy solutions, wind farms have emerged as a leading source of renewable power. However, the journey towards harnessing the power of wind is not without its challenges. The initial costs involved in building a wind farm are substantial. Furthermore, Operation and Maintenance (O&M) costs for wind turbines are a significant aspect of the overall economics of wind energy projects. According to WindFacts, O&M costs make up 20-25% of the total levelized cost per kWh produced over the lifetime of a turbine.
One of the most common reasons for wind turbine malfunctions is main bearing (MB) failure. The main bearing is responsible for supporting the turbine rotor, allowing it to rotate under its own axis while reducing friction between moving parts under high weight and variable loads. A study by the European Academy of Wind Energy found that MB failure rates can be as high as 30% over a 20-year lifetime, and identified it as the second most important reliability challenge behind gearbox issues.
When the main bearing fails on a wind turbine, it stops producing energy and, as a result, revenue. Its replacement requires the complete removal of the rotor, with the cost of the crane to perform the maintenance work at 10 to 20 times that of the bearing itself—especially for offshore wind farms.
As of August 2023, there are more than 90,000 wind turbine installations in the U.S., and an estimated 400,000 turbines worldwide, making main bearing failures responsible for for hundreds of millions of dollars in lost revenue.
Existing solutions are insufficient
While solutions based on vibration sensor data exist to detect main bearing failures with a high degree of accuracy, they have fundamental limitations that prevent them from being operationalized effectively. Specifically, without concrete estimates of remaining useful life (RUL), it isn’t clear how to prioritize among turbines with identified MB issues, or between turbines with MB issues and the rest of the maintenance backlog.
This uncertainty leads to either:
- A failure to prioritize replacements for impending MB failures
- Replacing the MB too early, thereby shortening its useful life
How Tagup is addressing the main bearing failure problem with machine learning (ML)
One of our customers is an operator of tens of onshore wind farms across the United States, Canada, and Mexico. The company has an installed capacity of approximately 6 gigawatts (GW) combined power output. Here’s how we solved their main bearing failure problem by leveraging Foresight, our critical event prediction and risk management ML software:
Main bearing failures are responsible for roughly half of the downtime events on the turbine class of interest (Siemens 2.3MW). When unexpected main bearing failures occur and require turbine shutdown, maintenance backlog and operational constraints make it difficult to find time to replace the bearing immediately. Rather than keeping the turbine shut down until the replacement can be scheduled, the company often de-rates the turbine, operating at a reduced power output to delay the overheating of the damaged bearing. Unfortunately, this “band-aid” solution is not ideal—material revenue is lost with reduced power output, and the bearing will not last indefinitely even when run in this low-power regime.
Tagup estimated, as a direct result of main bearing failure, nearly $820,000 in lost revenue over a 28-month period across three wind farms. Revenues lost on a per-turbine, annualized basis, were approximately $1,500.

The outcome and potential value at scale
Tagup leveraged its deep experience in time-to-event modeling, building a model to predict main bearing failures with two-to-six months of lead time, including best-in-class estimates of remaining useful life.
Given SCADA sensor data on 232 turbines across three wind farms trained on only 29 failure examples, Tagup’s model was able to predict eight imminent failures with perfect accuracy (all true positives identified, no false positives) and RUL predictions accurate to within 17 days of the actual failure date. Unlike existing solutions which require expensive vibration sensors that often don’t integrate into data workflows and fail to provide any RUL estimate, Tagup’s modeling approach allows engineers and technicians to effectively prioritize targeted maintenance actions, minimizing revenue loss across the fleet.
Deployed at scale, using our previous estimate of $1,500 per turbine in avoided revenue loss, Tagup could create more than half a billion dollars ($600 million) in global value for wind operators.
Contact us to improve the reliability of your industrial assets or learn more about our AI-driven solutions.

